AgriAdapt
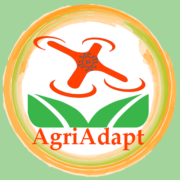
In AgriAdapt, we develop a context-adaptive deep neural network (DNN) compression mechanism that leads to reduced resource usage in drone onboard processing for weeds detection.
AgriAdapt introduces a novel dynamic resource-efficient machine learning technology to the domain of unmanned aerial vehicle (UAV)-based precision agriculture. Cameras mounted on unmanned aerial vehicles (UAVs) can provide a detailed picture of the fields, and deep neural network (DNNs)-based object recognition can help us understand the state of the land. However, the full potential of computer vision can be realized only if the processing happens directly on UAVs. This would enable UAVs to provide information, which could be used for real-time location-specific actioning, e.g. UAV could detect weeds, which an on-the-ground robot could immediately exterminate.
Within this project, the partership with the University of Ljubljana (UL) allowed to develop a context-aware neural network adaptation framework with dynamic slimming of the topology so that the memory and computational burden of the network adapts to the problem at hand. This translates to energy savings, as the amount of computation on the UAV is reduced in real-time with negligible loss of inference accuracy. The project was selected and supported by the SMART4ALL Innovation Action.
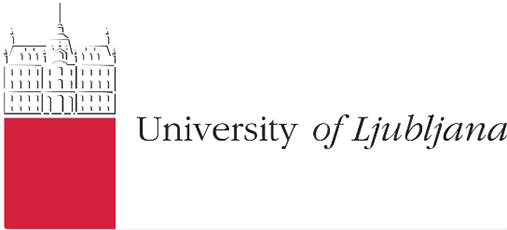
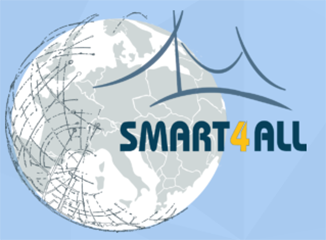